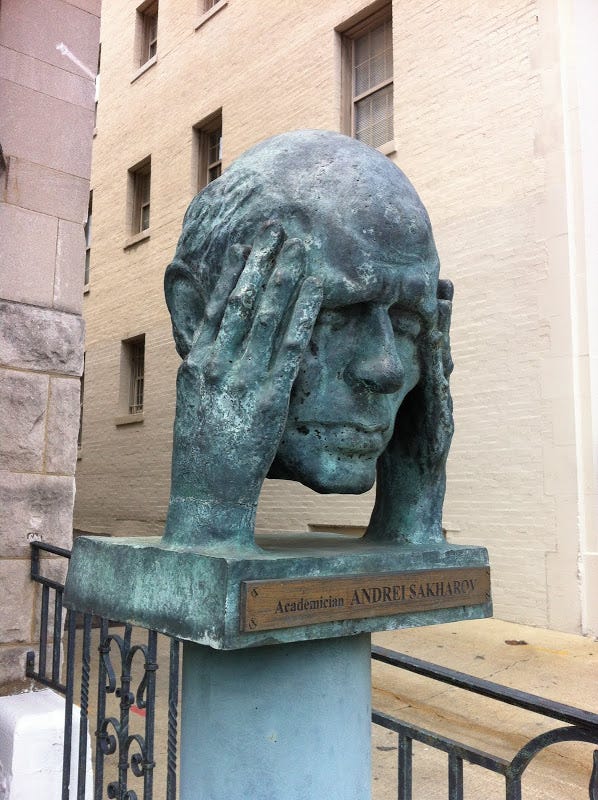
Authors
Chris Canal is a computer scientist with a background in machine learning and data science. He co-founded Wizio, a real estate software company, and is currently developing models that predict driver and customer behavior on Amazon's Last Mile team.
Connor Tabarrok is a civil engineer who finds the overlap of AI and governance interesting and important to the future of humanity. He currently works as a water resources consultant and writes a blog at alltrades.substack.com.
Overview
The development of Artificial General Intelligence (AGI) presents both large opportunities and large risks. By examining two global crises and world government responses, namely the Nuclear Test Ban Treaty and the Montreal Protocol, we create a general model of risk response and attempt to develop a general model of risk-mitigation policy. We survey possible public and governmental responses to AGI risk and recommendations for regulators.
We think the timeline of risk falls into 4 phases, with a “safety track” running in parallel.
Ideation
Research and Development
Technology Implementation
Policy Implementation
We do our best to draw parallels to AGI development as of April 2023, and make predictions and recommendations based on our analysis of the past. In general, we are optimistic that humanity can coordinate to mitigate this risk as we have in the past, but we are not so naive to think that humanity couldn’t mismanage the situation. A key idea for readers to carry through the piece is that the more revolutionary the technology, the more can be lost by regulatory mis-calibration in either direction (under or over regulated).
IDEALIZED RISK-RESPONSE MODEL:
Introduction
Our course of action in the present will determine if our reality will diverge into utopia or into doomsday. In the most utopian outcome, we would be able to fully harness the power of AI and subsequent technological improvements with little to no costs of adoption. By minimizing inefficiencies in AI regulation, we can approach this outcome. In the most doom-ish of possible outcomes, AI gains capabilities at a rate much faster than we are able to give feedback or make course-corrections, and humanity as we know it is destroyed.
A model of this problem already exists in policy. To determine the correct course of action, one cannot only look at the price of inaction (in this case, letting global temperature rise). One must also consider the price of policy, and only by knowing the relationship between the two can we determine the most optimal solution mix. This idea is illustrated in the graph below, from climate scientist Bjorn Lomberg. The graph shows the cost of global temperature control policy and identifies the most optimal amount of allowable temperature rise given the costs of both action and inaction.
While this point does exist, it is not so easily agreed upon as the above graph may lead you to believe. And, as the diversity of viewpoints grows, it becomes harder and harder to reach consensus on the degree to which we should trade off progress vs risk. This problem is exacerbated by the inequity with which specific costs are borne to stakeholders in the policy process.
This is a unique era, because the bureaucratic mechanism governing progress is more powerful than ever before. If today’s laws had been around at the time of the invention of the wheel, it may very well have never passed the safety board’s review process. We find it hard to discount the potential futures that will be lost if we over-regulate revolutionary technology, considering that we are the product of such technology ourselves, and to apply our modern standards retroactively would easily set us back hundreds of years and billions of lives.
We will explore past catastrophes that humanity has successfully steered away from through coordination. From these examples, we can create useful abstractions and lessons to strategize for preventing unaligned artificial intelligence.
Case 1. Nuclear Test Ban Treaty
One of humanity's most notable policy successes over an existential risk is the Comprehensive Nuclear-Test-Ban Treaty (CTBT). In an attempt to make sense of a complex timeline, we’ve overlaid the events leading up to the comprehensive test ban treaty (CTBT) with what we think of as the four phases of an existential risk technology.
Four Phases of Existential Risk Tech
Ideation
Engineering
Technology Implementation
Policy Implementation
We chose these four phases because they fit the progression of nuclear risk and AGI quite well. Examining the timeline of these risks revealed how little time there might be between the first large-scale experiment and possible catastrophe. Most people are surprised to learn that only 21 days passed between the first successful nuclear weapons test, the trinity test, and the first nuclear detonation over a city: Hiroshima. These phases also seemed to indicate that the slow development of policy and safety procedures might have influenced overly strict nuclear policy that resulted in the world not being able to move completely to clean nuclear energy. We want as many benefits from AGI as possible without an unreasonable risk of extinction. From studying nuclear weapon development and the evolution of policy we have the following takeaways.
Takeaways from Examining the History of Nuclear Weapons
Humanity might decrease risk most by employing the top minds to logically prove that a technology is safe prior to creating large scale experiments.
Restricting access to information and materials (supply chain) is critical to reducing risk.
Effective Policy takes iterations / time, and technology moves faster than policy or safety, but public fear can act as a catalyst for speeding up policy development.
Policies that reduce risk often incur some cost to the technologies benefits.
Cultural, Policy, Market, and Logistic forces can deter existential disasters from occurring.
Once a powerful technology is proven safe there is still the problem of aligning the controllers of that powerful technology with humanity's best interest.
Provably Safe
The importance of ensuring the safety of a technology before it is scaled or implemented widely is exemplified by the existential safety concerns first raised by Robert Oppenheimer and Hans Bethe. Prior to the first nuclear bomb test, Oppenheimer worried about the possibility of a nuclear chain reaction igniting the Earth's atmosphere. This concern led to multiple scientists working on the relevant calculations, ultimately concluding that such an event was "incredibly impossible." This demonstrates the value of engaging top minds in logically proving a technology's safety prior to conducting large-scale experiments. In the context of AGI, adopting a similar precautionary approach might involve employing the top scientific minds like Hinton, Terance Tao, or Bengio to prove the safety of backpropagation neural networks. This could help prevent unintended consequences or misuse of the technology, thus minimizing the risk to society and the environment.
Restricting Access
The importance of controlling access to information and materials related to potentially dangerous technologies is exemplified by the Manhattan Project and the Non-Proliferation Treaty (NPT). By restricting nuclear weapon theory and development to a select group of top minds during the Manhattan Project, safety calculations were completed prior to major tests, reducing the risk of catastrophic accidents. Additionally, the NPT, implemented in 1970, limited the number of nations possessing nuclear weapons, effectively constraining the development of a global supply chain for enriching nuclear materials. While this restriction may have slowed the widespread adoption of nuclear energy and its potential benefits in reducing pollution and emissions, it also significantly increased the barrier to entry for the technology. Consequently, this limited the chances of a malicious actor obtaining a nuclear bomb, illustrating the importance of balancing safety concerns with technological advancements. Similarly, implementing strict supply chain controls for GPU clusters and information access protocols for blackbox AGI development can help prevent misuse or malicious exploitation of the technology, thus reducing the overall risk associated with it.
Policy iterations over decades
The inherent challenge of keeping pace with rapidly advancing technology is highlighted by the history of nuclear policy and the time it took for effective regulations to be implemented. While the Manhattan Project produced a working nuclear weapon in just a few years, the development of comprehensive policies took much longer. The unsuccessful Baruch Plan, proposed in 1946 as the first nuclear regulatory policy, did not immediately result in any meaningful international agreements. Public fear acts as a catalyst for speeding up policy development, as evidenced by events in the 1950s and 1960s. The Castle Bravo nuclear test, which sickened Japanese fishermen, the St. Louis Baby Tooth Study, and the 1951 "duck and cover" protection method, all stirred widespread concern and prompted politicians to implement policies such as the Limited Test Ban Treaty in 1963. It took another 33 years and many policies before a comprehensive ban on this dangerous technology was written and signed by most countries.
In the context of AGI, understanding the relationship between public perception, policy development, and technology advancement is crucial. Humanity might suffer from an intelligence explosion before we can figure out the proper safety guard rails for developing advanced AI. Close collaboration between policymakers and researchers is necessary for anticipating potential risks and proactively addressing them, ensuring that AGI development remains aligned with societal values and safety requirements. It might be necessary for an extreme amount of public concern to catalyze policy implementation and iteration that could match the speed of AI capabilities research.
The Cost of Safety
An important consideration when implementing policies to reduce existential risk is the potential trade-off between risk mitigation and technological benefits. The Non-Proliferation Treaty (NPT), while successful in limiting the number of nations possessing nuclear weapons, has also restricted access to clean nuclear energy for many countries. This constraint has undoubtedly contributed to increased pollution and could be a major factor in the ongoing challenge of addressing climate change. While it is essential to protect society and the environment from the harmful effects of powerful technologies, striking a balance between risk reduction and promoting technological advancements is crucial. In the context of AGI, finding this balance will ensure that the benefits of the technology are maximized without incurring unreasonable risks or hindering its potential positive impact on technological progress.
Types of Deterrents
The history of nuclear weapons demonstrates the complex interplay of cultural, policy, market, and logistic forces in deterring existential risks. The Nuclear Test Ban Treaty, coupled with the pervasive fear of nuclear conflict in the public zeitgeist, shifted the Overton Window, raising the threshold of irrationality required for a world leader to consider launching a nuclear strike. This change in public perception and international norms serves as a cultural deterrent to the use of nuclear weapons.
Logistical deterrents like nuclear proliferation efforts have made the process of acquiring and deploying fissile material refineries more difficult, reducing the number of actors with the resources and capabilities to create nuclear weapons. Additionally, the inherent rarity and expense of fissile materials serve as a market deterrent for nuclear weapons development, further limiting their spread.
Policy plays a crucial role in shaping these deterrents, as it can directly impact culture, logistics, and market dynamics. By understanding and leveraging these dynamics, policymakers can develop more effective strategies for mitigating the risks associated with AGI, while still fostering its positive potential for society. The raw ore of AGI is supercomputing clusters and datacenters which currently have no guard rails or restrictions for AI capabilities research and usage. Restricting these resources in some way would likely reduce risk.
Human Alignment
The challenges of aligning powerful technologies with the greater good are further complicated by the diversity of human values, which are shaped by factors such as genetics, culture, and upbringing. Considering the needs and perspectives of both current and future generations, as well as accounting for potential value drift, can make it difficult to establish a universally acceptable framework for guiding technology.
Historical examples, such as the Cold War and the more recent emergence of North Korea as a nuclear nation, along with the potential nuclearization of Iran, demonstrate the complexities and risks associated with multiple players in the nuclear weapons game. As the number of players increases, the global landscape becomes more unstable, and the probability rises that a malicious or unstable individual could gain control of a nuclear nation or its arsenal.
These same problems—aligning human values and preventing the misuse of powerful technologies by those with anti-human sentiment—will continue to be relevant and potentially even more salient in the context of AGI. Learning from the experiences of nuclear policy, it is crucial to establish mechanisms that ensure the development and application of AGI are steered towards the greater good, while carefully considering the diverse values and interests of humanity in order to minimize potential conflicts and risks.
Conclusion
The history of nuclear weapons and policy development, as illustrated by the Comprehensive Nuclear-Test-Ban Treaty (CTBT), offers valuable insights into managing existential risks posed by powerful technologies. By examining the four phases of existential risk technology (Ideation, Engineering, Technology Implementation, and Policy Implementation), we can better understand the progression of nuclear risk and AGI, as well as the pressing need for effective policy and safety procedures.
By learning from the successes and challenges of nuclear policy, such as the CTBT, we can better prepare for the potential risks and opportunities associated with AGI. Establishing a delicate balance between safety, technological advancements, and diverse human values will be crucial in guiding AGI development and ensuring that its benefits are maximized for the greater good, while minimizing conflicts and risks.
Case 2. The Montreal Protocol
Ideation
CFCs were first synthesized in the 1890s, by Belgian Chemist Frederic Swarts, his work mainly focused on the process of synthesis, and the properties of organic fluorine bonds, and was relatively agnostic to how CFCs could be used. Later development by Thomas Midgley Jr. (Who also helped create leaded gasoline) would see the CFC synthesis process industrialized, and the first CFCs produced on a large scale.
R&D
Midgley would go on to use CFCs to replace the toxic chemicals previously used in air conditioners and refrigeration systems, and market his new refrigerant as Freon under the DuPont chemical company umbrella.
Technological Implementation
CFCs found a broad set of uses after their industrial/commercial debut in the 1930s and were widely employed as refrigerants, propellants, fumigants, industrial solvents, and fire extinguishers. CFCs had an appealing set of useful properties, (namely having low toxicity, low reactivity, and low flammability, as well as a low boiling point) and were simple molecules, relatively easy to manufacture. However, their stability, the very quality that was thought to make them safe, is what allowed them to reach the upper atmosphere without decaying. CFCs have a half life of more than a hundred years, giving them time to spread to the far reaches of the upper atmosphere by means of diffusion, and once there, CFCs are exposed to large amounts of UV radiation, causing an ozone depletion cascade to occur as detailed below. Ozone blocks UV radiation, which protects us from cancer. In the 1970s, scientists at UC Irvine began research into the impact of CFCs on the upper atmosphere, finding the link between CFCs degradation in the atmosphere and the process of ozone depletion, and testified before congress on the matter in 1974. Significant funding was awarded to investigate the matter further and their findings were confirmed by 1976. Alarm bells rang out in 1985 when large-scale depletion of the ozone layer over Antarctica was noted by explorers with the British Antarctic Survey, giving birth to the concept of the “ozone hole”. The hole's existence was confirmed by NASA satellites shortly thereafter. In 1995 the principal investigators from UCI Molina and Rowland as well as Paul Crutzen, would be awarded the Nobel Prize for their findings.
Policy Implementation
Progress in banning CFCs was slowed in the U.S. legislature by lobbyists from chemical companies like Dupont, who insisted on scientific rigor to confirm the findings rather than swift unilateral action from the U.S. government, in the hopes that multilateral action would be plagued with failures of coordination. Critically, the Protocol also included a funding scheme to support and finance the conversion of existing manufacturing processes, train personnel, pay royalties and patent rights on new technologies, and establish national ozone offices in all signatory countries.
In 1985, 20 of the largest CFC producing nations would sign the Vienna Convention, which laid out a framework for emissions reduction negotiations. Since then, over 197 countries have ratified the agreement, and it has gone through multiple amendment cycles, which have been broadly adopted.
The top two graphs in the figure below show the progress towards substituting CFCs with HCFCs (hydrochlorofluorocarbons), which are less harmful to the ozone layer but have recently been linked to global warming, having an effect possibly as potent as 10,000x the equivalent amount of carbon dioxide.
Thanks to revisions, HCFCs are due to be phased out of production by 2030 by adherents to the Montreal Protocol, and are planned to be replaced with HFCs (hydrofluorocarbons). The figure in the bottom right of the 4 graphs shows the slowing levels growth and eventual decline of halon levels, this is due to fire systems slowly releasing the chemical upon use/decay, but new systems rarely use halons anymore so this trend is set to continue steadily.
Policy Compliance & Success
Compliance to the Montreal Protocol is less than stellar. Throughout the 90s, Russia sold large quantities of CFCs to EU markets over the black market. U.S. production and consumption of CFCs was covered up by fraud and regulatory gymnasts evading lumberingly slow enforcement mechanisms, and underground markets are known to have existed in Taiwan, Hong Kong, and Korea. In addition, satellites over East Asia picked up significant increases in CFC production and emission around North Korea and China’s Shandong Province in 2012, indicating that countries are willing to cheat to get ahead in industry.
Despite spotty enforcement and the obvious free rider problems that the Montreal Protocol presents, it has been largely successful. Signatories have eliminated 98% of ozone depleting chemicals, which by some estimations will prevent 443 million cases of skin cancer.
However, employing this policy model in an AI/alignment risk scenario presents a significantly harder challenge, as 98% compliance is still a failure in an AI risk scenario, and the short term gains of being the only party with access to powerful AI are much greater than CFC use.
Montreal Protocol Takeaways
In our estimate, the most important and underrated aspect of the Montreal Protocol was the funding and incentives for scientists to develop appetizing alternatives to CFC use, allowing the world to narrow the gap in competitiveness between CFC users and abstainers enough that the remainder could be bridged with social and diplomatic pressure. Essentially, the policy’s effect of slowing ozone depletion down while also speeding up development of alternatives is what allowed us to fix the problem.
This solution case is distinct from the current state of nuclear risk mitigation. There has been no technological solution to nuclear risk thus far, and countries still control nuclear stockpiles large enough to destroy humanity. Thankfully, the policy solution in place has continued to buy time for solutions to be developed, but for now, humanity remains at risk. In the case of ozone depletion, policy funded the creation of safer alternatives to ozone depleting chemicals, to the point where the risk to the ozone layer is completely mitigated. This scenario represents the most optimal outcome of AI policy, where the imposed incentives shift funding, interest, and brainpower away from the capabilities space and towards the alignment/safety research space, reducing the risk to the species until progress in safety meets or exceeds progress in capabilities, and a “safe” substitute good is developed.
Substitute Goods for Agentic AI
The Nuclear Test Ban Treaty and the Montreal Protocol exemplify how policy can mitigate technological risks by restricting access to harmful technologies or promoting substitutes. These solutions offer valuable insights into AI governance.
Current regulatory frameworks can adapt to manage AI usage, as evidenced by existing licenses and security clearances in biotech, aerospace, and nuclear sectors. Though malicious use on the fringes may persist, benevolent complementary technologies, akin to antivirus software, can counterbalance potential threats.
However, devising substitutes for agentic AI proves more challenging. An AI whose outputs are sufficiently tied back to its own inputs can be placed on a spectrum of agency, and there exists a threshold where an AI can be considered a stakeholder in policy considerations. We’ll refer to this as “agentic AI”, but this is just shorthand for “sufficiently agentic AI”. To deter its development or application, alternatives must offer enticing benefits while diminishing agentic AI's relative appeal. Three potential substitutes are:
Pharmaceutical Neurogenesis: Enhancing cognitive abilities with pharmaceuticals narrows the gap between human and agentic AI intelligence, reducing agentic AI's allure while mitigating certain risks.
Brain-Computer Interfaces: Such interfaces could augment human intellect with silicon-based capabilities, rendering agentic AI less attractive. However, the extent of integration might dictate our vulnerability to agentic AI-related disasters.
ASI Toolboxes: Developing advanced specialized intelligences (ASI) that enable human-AI collaboration to approach agentic AI-level performance could level the playing field, decreasing the marginal value of agentic AI.
These substitutes, while mitigating risks, still expose us to the potential misuse of powerful tools, reminiscent of the challenges faced in the Nuclear Test Ban Treaty. However, given the substantial precedent in managing such risks, this approach appears more favorable than alternatives that lack substitutes, such as a Butlerian Jihad or development bans.
By shifting focus from agentic AI development to cultivating substitutes, we can minimize risk by reducing the incentive for pursuing hazardous technologies, while arming ourselves with potent tools in case such technologies emerge.
Case 3.
AI Development
While there is disagreement on when AGI might come about, one thing is for sure: rate of development of AI capabilities is accelerating. The graphic above shows a timeline of important events in AGI development. You can see that the frequency of milestone events has been increasing as we approach and then pass the current day. This acceleration towards the unknown future is something that we can measure in terms of computational power available, the efficiency with which we harness that power, and the scale at which that power has been deployed. We know this acceleration is occurring not only through these discrete measurements, but also through lived experience. An AI researcher in 2012 knew almost as much as an AI researcher in 2017, but the gap between 2017 knowledge and 2022 knowledge is comparatively gargantuan. In the next graphic, we’ll examine the spread of opinions among experts in the AI field. Here too, the consensus can be summed up by the word “acceleration”.
The graphic above shows how AI experts feel about the relevant timeline for AGI-risk. (More info here)
AI Risk Profile and Policy Ingredients
When considering the risk profile of AI technology, it is important to distinguish between AI as a tool, and agentic AI. In the generalized risk response model, AI tools like LLMs have already reached the technological development stage, whereas an agentic AGI is still in the Ideation and R&D phases. We don’t know if further development in AI will be limited to the development of better tools, or if it will result in a new and powerful agent on the scene. This represents an interesting parallel to atomic research and the trinity test specifically. We won’t know if agentic AI will kill us all until we take the risk and develop it, just like the Manhattan project scientists didn’t know if the atmosphere would ignite during the Trinity test. AI policy should seek to align both humans equipped with new AI tools and a potentially agentic AI.
What are the ingredients that result in risk response policy? Observing past precedents, we have identified 4 pre-conditions that seem to make successful risk-response more likely .
Fear- The public acknowledges to some degree the threat that the risk poses to them.
Focus- The risk is a priority in the public’s mind
Feasibility- The public believes that a policy solution is possible, and therefore worth pursuing
Champion- The public has a conduit into which they can channel their support for risk response policy. This person or persons represents policy action in the space and advises politicians or holds political power themselves.
AI Policy Grab Bag Analysis:
To survey some possible policies (there are lots) that could be enacted in the face of AI risk, we chose two extreme policies on differing corners of the cost/success coordinate grid and considered 7 others that bridged the gap between them. These anchor policies were the Windfall Clause, and a Butlerian Jihad. We defined our success scenario as:
“AI risk reduction policy is enacted that ensures safety research outpaces capabilities growth while leaving the least progress on the table”
We think that this a good AGI policy probably consists of a few key parts
Narrow the total cohort of AI capabilities researchers, excluding a larger absolute number of high-risk actors (Yud refers to them as “Human disaster monkeys”).
Create and Subsidize “safe” AGI alternatives that act as substitute goods
Carefully partition research resources among a smaller number of more cautious people.
Eliminate the race to the bottom dynamic.
Establish a stable scientific environment that balances progress and caution.
Windfall Clause
The Windfall Clause is a policy proposal for an ex ante commitment by AI firms to donate a significant amount of any eventual extremely large profits garnered from the development of transformative AI. By “extremely large profits,” or “windfall,” it means profits that a firm could not earn without achieving fundamental, economically transformative breakthroughs in AI capabilities.
Properly enacted, a policy making the Windfall Clause mandatory could address several potential problems with AI-driven economic growth. The distribution of profits could compensate those rendered faultlessly unemployed due to advances in technology, mitigate potential increases in inequality, and smooth the economic transition for the most vulnerable. However, the windfall clause only aligns human actors, and does so weakly, with very little means of enforcement once a firm becomes dominant via some breakthrough. For this reason, we rated it as the lowest-cost, lowest success policy.
GPU Hegemony Trimming
This policy represents a possible international treaty aimed at preventing any one country or entity from dominating processing power, and could potentially contribute to a more equitable balance of power in the digital age. By drawing upon historically precedented international collaboration between great power rivals, this treaty could seek to promote and maintain a balance among nations, preventing any single actor from achieving excessive control over global digital resources. However, the effectiveness of such a treaty could be limited by differing national interests and the inherent difficulties in monitoring and enforcing compliance, as was the case with the League of Nations. Moreover, the costs of implementing such a treaty could include stifling technological innovation, as nations might be hesitant to invest in advanced computing capabilities if they are subject to strict international limitations. We thought that because of the historical precedent for international governance and the interest of the group as a whole in preventing the emergence of a hegemonic power, that this policy would be relatively easy and low cost to implement, but still rated it as a low-success policy, because it only works to level the playing field between human-entities, but does not really help to prevent the emergence of an unaligned intelligence.
Universal GPU Physical Kill Switch
The likelihood of successfully slowing AI capabilities growth through this method is uncertain, as the global nature of the tech industry would necessitate widespread cooperation and compliance, however, were compliance achieved, it could function as an important reactive measure to the detection of a nefarious AI. The existence of such destructive failsafes may also discourage the development of AIs in general and cause the ones that are developed to be more narrowly interpretable, for fear of being miscategorized as nefarious. Installing a destructive failsafe on every GPU and manufacturing new GPUs with a similar feature could lead to increased production costs and potential disruption in the supply chain. Additionally, the implementation of such a failsafe could result in unintended consequences, such as accidental activation causing widespread damage to critical infrastructure and economic loss. Furthermore, this approach may inadvertently incentivize a black market for GPUs without failsafes, potentially exacerbating disparities in AI capabilities between nations and entities. Because it would involve so many changes in the industrial chain of one of the most productive tools at humanity’s disposal, and the likelihood that the switch could be co-opted by hackers or triggered via false-alarm, we rated this as a high cost approach, with a relatively low rate of success, due to its reactive rather than proactive nature.
Air Traffic Control for GPU clusters
This policy is essentially a Bostrom Panopticon, but only imposed on operations above a certain compute threshold, akin to Air Traffic Control. This could potentially help regulate the development and deployment of advanced AI systems, and make sure that the ones deployed are both telegraphed in advance and not developed in a bubble or echo chamber. However, the likelihood of success would largely depend on global cooperation and adherence to the monitoring system's guidelines, which could prove challenging given the wide range of stakeholders involved. The costs of establishing such a system could be significant, as it would require extensive infrastructure, resources, and personnel to effectively monitor and enforce compliance. Moreover, privacy concerns and potential misuse of gathered data could arise, creating additional challenges and potential backlash from both the tech industry and the general public. We thought that if implemented correctly, this could be quite successful at preventing risky research from happening at a large scale, but given the human effort and international coordination/cooperation this would require, we also rated it as high cost.
Security Clearances for AI Capabilities people
This policy is similar to the ATC policy but instead of only monitoring GPU clusters, you would restrict access to those clusters and related information to a select group of vetted individuals, similar to security clearances in the defense and aerospace sectors. It comes at a high cost of implementation, as vetting processes are long and exclusionary, but parallels already exist in the defense and intelligence branches of the government, and could conceivably be extended. Both safety and capabilities work would be slowed by this policy, but snipping the cohort of people with access to ML tech based on risk tolerance would disproportionately exclude the high-risk members of such a cohort, and thus make the field as a whole more insulated to any one researcher taking on huge risks in a “race to the bottom” dynamic, with regards to safety, in a bid to be first to an agentic AI. Similar to the ATC idea, this would be a high cost solution, but by governing the human user rather than the GPU cluster, and incorporating the rigorous vetting process would add an extra layer of protection by excluding not only bad research, but also bad actors. Thus, we rated it as roughly equal cost (it’s cheaper to just deny lots of applications, but more progress is lost here), but significantly more likely to slow AI capabilities.
No cloud data centers, host internet on distributed network of personal devices
This item represents a policy that promotes a decentralized networking of personal and home devices, as opposed to centralized data centers. Implementing such a policy could potentially reduce the risk of a hostile algorithm running rampant and gaining control over significant computing resources. Decentralization could also increase overall system resilience and democratize access to computing power. However, implementing such a policy might introduce security and privacy concerns, as personal devices could be more vulnerable to attacks or unauthorized access. Additionally, the effectiveness of this approach in slowing AI capabilities growth would depend on widespread adoption and overcoming potential technical challenges, such as ensuring consistent performance and reliability across a diverse range of personal and home devices. We thought that because it would reduce the likelihood that a superintelligence could run rampant in a datacenter, it could be pretty effective at slowing AI risk timelines. However, this policy would mean a large restructuring effort of the internet, which would undoubtedly have high costs.
Nick Bostrom's Surveillance Panopticon
A policy inspired by Nick Bostrom's idea of a surveillance panopticon would involve constant monitoring and evaluation of AI development to prevent the emergence of misaligned AI systems. This approach could potentially identify and mitigate risks associated with powerful AI before they pose significant threats. However, such a policy would raise privacy and ethical concerns, as constant surveillance may infringe on individual freedoms and stifle innovation within the field. Furthermore, the effectiveness of this policy would depend on international cooperation, comprehensive monitoring capabilities, and the ability to enforce compliance across various organizations and countries.
Yudkowsky Inquisitions
This policy represents a slippery slope interpretation of Yudkowskian recommendations in the face of AI risk. Its main tenets would include a ban on corporate scale AI use, the destruction of every large scale data center, and confiscation of all GPUs except for a small share of AI researchers that can pass a psych screen. Rogue researchers who are deemed misaligned would be systematically rooted out and disenfranchised of their equipment. Knowledge of linear algebra is restricted only to a select clerical class who have completed the prerequisite reading. We thought that this policy would be slightly more costly and slightly more effective than Bostrom’s Panopticon, if implemented to similar degrees, but would slow safety research greatly.
Butlerian Jihad & return to Mechanical Computing
The most extreme policy we considered is a total destruction of all computing machinery with the exception of mechanical computers, a la the “Butlerian Jihad” from the popular science-fiction series Dune. While it would certainly buy time to do safety research and prevent tail-end actors from taking on too much risk, it would be extremely costly, set back progress by a significant amount, and likely slow the safety research itself. This policy was the highest in terms of both cost and effectiveness that we considered, although more different policies may present different tradeoffs.
Conclusion
There is a lot to learn from precedents when considering possible risk mitigation policies. Policy is a powerful tool to curb existential risk. However, all policy comes at a cost, both in its implementation, and in its effects. Considering these costs is key to policy that is both feasible and worth implementing. By examining the successes of the Nuclear Test Ban Treaty and the Montreal Protocol, we show that policy has the ability not only to delay the onset and minimize the magnitude of risk, but also to help spur technological inventions that eliminate the risk altogether. When examining the risk profile of AI, two distinct problems present themselves.
1.) How to align a potential agentic AI
2.) How to align humans with powerful AI tools
Parallels in our examples help to shed some light on these issues. With regard to nuclear weapons, our policy has been a powerful tool to instill opposed actors to a common goal of nuclear abstinence. We also see a parallel to the risk that a potentially unaligned agentic AI might present, when examining Arthur Compton’s worry that the Trinity test had the potential to ignite the atmosphere and bathe the earth in fire. To address this, he insisted on independent mathematical verification among Oppenheimer’s team that the chances of a chain reaction of atmospheric hydrogen were less than 3 in one million before he would proceed. Creating algorithms that are more interpretable is key to making sure the future is populated by verifiably aligned AI. With regard to the Montreal Protocol and CFCs, we see the power that policy has to bridge the gap between risky technology and safer alternatives, by imposing enough cost to spur transitions. Without the development of alternative technologies like HCFCs and HFCs, it’s unlikely that a CFC ban would have been effective in protecting global ozone. This same problem exists in AI safety. Without developing safe alternative technologies, policy can only act as a delaying measure against AI risk. Thus, it is critical that safety research match pace with, and eventually exceed, capabilities research.
We established a general model of risk-response policy, and explained where we are in the model with regards to different risks. With nuclear weapons, we are in the policy implementation phase, but safety technology lags far behind. Thanks to our policy, we are able to delay the risk scenario of global destruction, but safety technology has not progressed to the point where that risk is mitigated. With regards to the Montreal Protocol, we have developed safe alternatives to risky technology, and are in the process of adopting those alternatives on a global scale, with HFCs thought to be on track for near global adoption by 2030. With AI, we are beginning to develop “safe” versions of some models, like consumer grade LLMs which refuse to fulfill requests deemed dangerous by their developers, but agentic AI presents a problem similar to that of the Trinity test, where iteration is extremely costly if the bad outcome of the risk scenario is encountered. For this reason, the confidence researchers have that the tool they are developing is either nonagentic or agenetic and aligned, needs to be extremely high, similar to Compton’s threshold of 3-in-1-million.
In addition to our generalized risk model, we also identified the key parts of risk response policy, and surveyed the space of policy ideas. These tools can help researchers and regulators guide us towards a better future. Success may take many forms, from a substitute good obsoleting AI and bypassing that risk, to a regulatory solution that simply extends timelines indefinitely. There probably won’t be a discrete event to point to as the instance “alignment” was solved. AI alignment is a complex and daunting landscape of ideas, with no expert consensus. If you found any of the ideas presented here valuable, we encourage you to incorporate them into your understanding and share with others. It’s estimated that <1000 people currently work directly on AI ex-risk (link), so if you're reading this, make yourself heard! Your ideas and insight have the potential to change minds and contribute to the field! We look forward to reading them.
This has so much good data
Substitute development is an underexplored risk reduction path-- good for you for highlighting it here. If we didn't have effective alternative refrigerants, we'd almost certainly still be using CFCs.
But it seems to me that the most important way the AI risk debate differs from previous such debates, including nuclear and ozone-destruction risk, is that it's not based on any direct evidence of harm. Nuclear test ban treaties were passed because we knew from terrible experience how destructive nuclear weapons could be; the Montreal Protocol came through because we had clear physical evidence of the ongoing harms of the ozone hole. All AI alarmists have are thought experiments and science-fiction scenarios. And the argument that we can't wait for direct evidence of harm because then it will be too late has a very bad history of being used to justify destructive, overreaching preventive actions: think of GW Bush justifying the disastrous Iraq invasion on the grounds that "we can't let the smoking gun be a mushroom cloud."